14 min to read
Setting up GPU Server on Google Cloud Platform
A complete guide to deploying and configuring NVIDIA A100 GPU instances on GCP
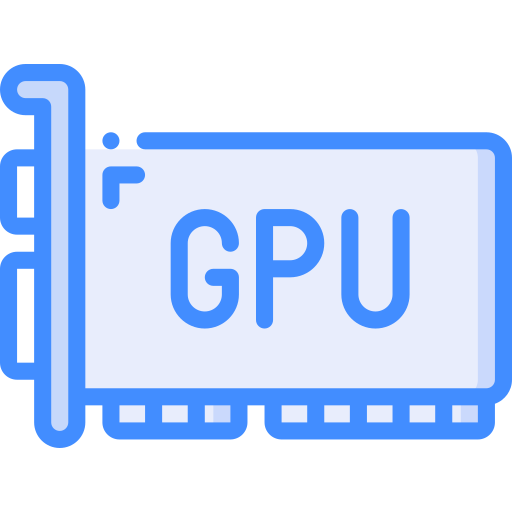
Overview
Google Cloud Platform (GCP) offers powerful GPU-accelerated computing instances that are ideal for AI/ML workloads, scientific computing, and rendering applications. This guide focuses on setting up and optimizing NVIDIA A100 GPU servers, which represent some of the most powerful GPU resources currently available in the cloud.
The NVIDIA A100 offers exceptional performance for deep learning training and inference, with variants including the 40GB and 80GB memory configurations. This guide walks through the entire process from checking GPU availability, requesting quota, deploying via Terraform, installing drivers and frameworks, to running popular AI applications.
Specification | A100 40GB | A100 80GB |
---|---|---|
GPU Memory | 40GB HBM2 | 80GB HBM2e |
Memory Bandwidth | 1.6 TB/s | 2.0 TB/s |
FP32 Performance | 19.5 TFLOPS | 19.5 TFLOPS |
Tensor Cores | 432 | 432 |
CUDA Cores | 6,912 | 6,912 |
TensorFloat-32 (TF32) | 156 TFLOPS | 156 TFLOPS |
GCP Machine Types | a2-highgpu-* | a2-ultragpu-* |
GPU Availability and Quota
Checking GPU Availability by Region
Before deploying a GPU instance, you need to verify which GPU types are available in your preferred region and zones. The availability varies by region, and not all zones support all GPU types.
Official Documentation
Using gcloud CLI to Check Availability
# Check A100 availability in Seoul region
gcloud compute accelerator-types list --filter="name:nvidia-tesla-a100 AND zone:asia-northeast3"
NAME ZONE DESCRIPTION
nvidia-tesla-a100 asia-northeast3-a NVIDIA A100 40GB
nvidia-tesla-a100 asia-northeast3-b NVIDIA A100 40GB
# Check A100 availability in all regions
gcloud compute accelerator-types list | grep a100
nvidia-a100-80gb us-central1-a NVIDIA A100 80GB
nvidia-tesla-a100 us-central1-a NVIDIA A100 40GB
nvidia-tesla-a100 us-central1-b NVIDIA A100 40GB
# ... more output ...
Requesting GPU Quota Increase
By default, GCP accounts have limited or no GPU quota. To use GPUs, especially high-end models like the A100, you need to request a quota increase:
- Navigate to IAM & Admin > Quotas & System Limits in the GCP Console
- Filter for “NVIDIA A100” or specific quota names
- Select the region(s) where you need quota
- Click “EDIT QUOTAS” and enter your requested limit
- Submit and provide business justification if prompted
Important Resources:
- Be specific about your use case (AI training, inference, scientific computing)
- Request quota in regions with known availability
- Specify timeline and duration of GPU usage
- For large quota requests, be prepared to provide business justification
- Allow 1-2 business days for quota approval
GPU Pricing
GPU pricing varies significantly by type, region, and commitment level. Always check the current pricing before deployment.
Official Pricing Documentation
NVIDIA A100 Pricing Example (as of 2025)
Machine Type | GPU Configuration | Monthly Cost | Daily Cost |
---|---|---|---|
a2-highgpu-1g | 1x A100 40GB | ~₩3.95M | ~₩120K |
a2-highgpu-2g | 2x A100 40GB | ~₩7.9M | ~₩240K |
a2-highgpu-4g | 4x A100 40GB | ~₩15.8M | ~₩480K |
a2-ultragpu-1g | 1x A100 80GB | ~₩6.2M | ~₩205K |
a2-ultragpu-2g | 2x A100 80GB | ~₩12.4M | ~₩410K |
a2-ultragpu-4g | 4x A100 80GB | ~₩24.8M | ~₩820K |
Cost Optimization Strategies
- Spot Instances: Use preemptible VMs for interruptible workloads (60-91% discount)
- Committed Use Discounts: 1-year or 3-year commitments for predictable workloads (20-57% discount)
- Right-sizing: Select appropriate GPU count based on actual needs
- Resource Scheduling: Create startup/shutdown schedules for non-production environments
- Monitoring: Set up budget alerts and utilization monitoring
Infrastructure Deployment with Terraform
Terraform allows for declarative, version-controlled deployment of GPU instances. Below is an example configuration for an A100 GPU server:
## ai_server ##
resource "google_compute_address" "ai_server_ip" {
name = var.ai_server_ip
region = var.region
}
resource "google_compute_instance" "ai_server" {
name = var.ai_server
machine_type = "a2-highgpu-2g" # a2-ultragpu-2g = A100 80G 2 / a2-highgpu-2g = A100 40G 2
labels = local.default_labels
zone = "${var.region}-a"
allow_stopping_for_update = true
tags = [var.nfs_client]
boot_disk {
initialize_params {
image = "ubuntu-os-cloud/ubuntu-2204-lts"
size = 100
}
}
metadata = {
ssh-keys = "somaz:${file("../../key/ai-server.pub")}"
install-nvidia-driver = "true"
}
network_interface {
network = "projects/${var.host_project}/global/networks/${var.shared_vpc}"
subnetwork = "projects/${var.host_project}/regions/${var.region}/subnetworks/${var.subnet_share}-ai-b"
access_config {
## Include this section to give the VM an external ip ##
nat_ip = google_compute_address.ai_server_ip.address
}
}
scheduling {
on_host_maintenance = "TERMINATE" # Required for GPU instances
automatic_restart = true
preemptible = false # Set to true for preemptible instances
}
guest_accelerator {
type = "nvidia-tesla-a100" # nvidia-a100-80gb = A100 80G / nvidia-tesla-a100 = A100 40G
count = 2
}
depends_on = [google_compute_address.ai_server_ip]
}
Key Terraform Configuration Points
- Machine Type Selection:
a2-highgpu-*g
for A100 40GB (where * is the GPU count)a2-ultragpu-*g
for A100 80GB (where * is the GPU count)
- Boot Disk:
- Use Ubuntu 20.04 or 22.04 for best compatibility
- Allocate sufficient disk space (100GB+) for datasets and model weights
- GPU-specific Settings:
- Set
on_host_maintenance = "TERMINATE"
(required for GPU VMs) - Use
guest_accelerator
block to specify GPU type and count - Consider setting
metadata.install-nvidia-driver = "true"
for automatic driver installation
- Set
- Networking:
- Consider whether your workload needs an external IP
- Configure appropriate firewall rules for your services
GPU Server Setup and Configuration
After deploying the VM, you need to install drivers, CUDA toolkit, and other necessary software.
Verifying GPU Availability
First, check if the GPUs are properly attached to the VM:
# OS Version
lsb_release -a
# Confirm GPU hardware detection
sudo lspci | grep -i nvidia
sudo lshw -c display
Installing Drivers and CUDA Toolkit
GCP can automatically install NVIDIA drivers if you set the install-nvidia-driver = "true"
metadata. If you need to install manually:
# Install required packages
sudo apt update
sudo apt install -y ubuntu-drivers-common
sudo apt install -y nvidia-driver-535
sudo apt install -y nvidia-cuda-toolkit
Installing cuDNN
NVIDIA cuDNN is required for deep learning frameworks:
# Download cuDNN (requires NVIDIA Developer account)
# Example assumes you've downloaded the archive
tar xvf cudnn-linux-x86_64-8.6.0.163_cuda11-archive.tar.xz
cd cudnn-linux-x86_64-8.6.0.163_cuda11-archive
# Copy header files
sudo cp include/cudnn*.h /usr/include
# Copy library files
sudo cp lib/libcudnn* /usr/lib/x86_64-linux-gnu
# Set permissions and update library cache
sudo chmod a+r /usr/include/cudnn*.h /usr/lib/x86_64-linux-gnu/libcudnn*
sudo ldconfig
Verifying Installation
Verify that drivers, CUDA, and cuDNN are properly installed:
# Check NVIDIA driver
nvidia-smi
# Expected output example:
# +-----------------------------------------------------------------------------+
# | NVIDIA-SMI 535.104.05 Driver Version: 535.104.05 CUDA Version: 12.2 |
# |-------------------------------+----------------------+----------------------+
# | GPU Name Persistence-M| Bus-Id Disp.A | Volatile Uncorr. ECC |
# | Fan Temp Perf Pwr:Usage/Cap| Memory-Usage | GPU-Util Compute M. |
# | | | MIG M. |
# |===============================+======================+======================|
# | 0 NVIDIA A100-SXM... On | 00000000:00:04.0 Off | 0 |
# | N/A 32C P0 39W / 400W | 0MiB / 40960MiB | 0% Default |
# | | | Disabled |
# +-------------------------------+----------------------+----------------------+
# | 1 NVIDIA A100-SXM... On | 00000000:00:05.0 Off | 0 |
# | N/A 34C P0 41W / 400W | 0MiB / 40960MiB | 0% Default |
# | | | Disabled |
# +-------------------------------+----------------------+----------------------+
# Check CUDA version
nvcc --version
# Test cuDNN installation
cat <<EOF > cudnn_test.cpp
#include <cudnn.h>
#include <iostream>
int main() {
cudnnHandle_t cudnn;
cudnnCreate(&cudnn);
std::cout << "CuDNN version: " << CUDNN_VERSION << std::endl;
cudnnDestroy(cudnn);
return 0;
}
EOF
# Compile and run the cuDNN test
nvcc -o cudnn_test cudnn_test.cpp -lcudnn
./cudnn_test
# Expected output: CuDNN version: 8600
Setting Up NVIDIA Docker (Optional)
For containerized workloads, NVIDIA Docker allows GPU access from containers:
AI/ML Application Setup
With the GPU environment configured, you can now set up popular AI/ML applications.
Stable Diffusion WebUI
A popular interface for text-to-image generation:
# Clone repository and run installation script
wget -q https://raw.githubusercontent.com/AUTOMATIC1111/stable-diffusion-webui/master/webui.sh
chmod +x webui.sh
# Start the WebUI with remote access enabled
./webui.sh --listen --xformers
Access the web UI at http://<your-vm-ip>:7860
Kohya_ss
A tool for training and fine-tuning Stable Diffusion models:
# Clone repository
git clone https://github.com/bmaltais/kohya_ss.git
cd kohya_ss
# Run installation script
./setup.sh
# Start the UI with remote access
./gui.sh --listen=0.0.0.0 --headless
Access the web UI at http://<your-vm-ip>:7860
ComfyUI
A node-based UI for Stable Diffusion:
# Clone repository
git clone https://github.com/comfyanonymous/ComfyUI.git
cd ComfyUI
# Install dependencies
pip install -r requirements.txt
# Start the UI with remote access
python3 main.py --listen 0.0.0.0
Access the web UI at http://<your-vm-ip>:8188
PyTorch and TensorFlow Performance Testing
Test the GPU performance with standard benchmarks:
Performance Optimization
NVIDIA A100 Performance Tuning
- GPU Compute Mode:
sudo nvidia-smi -i 0 -c EXCLUSIVE_PROCESS # For dedicated single process
- Memory Clock Optimization:
sudo nvidia-smi -i 0 -ac 1215,1410 # Set memory and graphics clocks
- Persistent Mode (reduces initialization overhead):
sudo nvidia-smi -i 0 -pm 1 # Enable persistent mode
System-level Optimizations
- Disable CPU Power Management:
sudo apt install cpufrequtils sudo cpufreq-set -g performance
- I/O Optimization for dataset loading:
echo 'vm.dirty_ratio = 10' | sudo tee -a /etc/sysctl.conf echo 'vm.dirty_background_ratio = 5' | sudo tee -a /etc/sysctl.conf sudo sysctl -p
- Memory Configuration for AI workloads:
echo 'vm.overcommit_memory = 1' | sudo tee -a /etc/sysctl.conf sudo sysctl -p
Framework-specific Optimizations
- PyTorch:
torch.backends.cudnn.benchmark = True # Auto-tuner for convolutions torch.backends.cuda.matmul.allow_tf32 = True # Enable TF32 for faster training
- TensorFlow:
import tensorflow as tf tf.config.optimizer.set_jit(True) # Enable XLA JIT compilation mixed_precision = tf.keras.mixed_precision.Policy('mixed_float16') tf.keras.mixed_precision.set_global_policy(mixed_precision)
Monitoring GPU Usage
NVIDIA System Management Interface
The primary tool for monitoring NVIDIA GPUs is nvidia-smi
:
# Basic GPU status
nvidia-smi
# Continuous monitoring (updates every 1s)
nvidia-smi -l 1
# Show memory usage by process
nvidia-smi --query-compute-apps=pid,process_name,used_memory --format=csv
# Monitor specific metrics
nvidia-smi --query-gpu=timestamp,name,pci.bus_id,utilization.gpu,utilization.memory,memory.total,memory.free,memory.used --format=csv -l 5
DCGM (Data Center GPU Manager)
For production environments, NVIDIA DCGM provides more advanced monitoring:
# Install DCGM
sudo apt-get install -y datacenter-gpu-manager
# Start the service
sudo systemctl start dcgm
# Run DCGM diagnostics
sudo dcgmi diag -r 3
# Monitor GPU stats
sudo dcgmi dmon
Integrating with Prometheus and Grafana
For long-term monitoring and visualization:
# Install Prometheus NVIDIA exporter
git clone https://github.com/NVIDIA/dcgm-exporter.git
cd dcgm-exporter
make binary
sudo make install
# Start the exporter
sudo dcgm-exporter
# Configure Prometheus to scrape the exporter
# Add to prometheus.yml:
# - job_name: 'dcgm_exporter'
# static_configs:
# - targets: ['localhost:9400']
Best Practices and Security
- Choose appropriate GPU type based on memory requirements and computational needs
- Consider scalability - start small and scale up as needed
- Check zone availability before planning deployments
- Monitor usage patterns and adjust instance types accordingly
- Use preemptible instances for non-critical, fault-tolerant workloads
- Implement auto-shutdown for development environments
- Use committed use discounts for predictable workloads
- Configure firewall rules to restrict access to necessary ports only
- Use service accounts with minimum required permissions
- Enable OS Login for centralized SSH access management
- Apply security patches promptly
- Enable Virtual Private Cloud (VPC) Service Controls for sensitive workloads
- Install the latest NVIDIA drivers compatible with your workload
- Configure appropriate CUDA and cuDNN versions
- Monitor GPU utilization and memory usage
- Implement proper cooling and power policies
- Use mixed precision training where possible
- Use high-performance storage options for datasets (SSD, Local SSD)
- Implement data caching mechanisms
- Consider Cloud Storage with parallel transfers for large datasets
- Implement proper backup strategies for trained models
Comments